Artificial intelligence (AI) is rapidly transforming our world, and two of the most exciting areas of development are generative AI and predictive AI. But what exactly are these different types of AI, and how do they differ from each other?
Read on to learn exactly how these innovative technologies work and how they're being applied today. Let's dive in!
Key Differences Between Generative AI and Predictive AI
Generative AI and predictive AI are two branches of artificial intelligence with different capabilities. But all the buzz surrounding these terms, it's easy to get confused about what they are and how they differ from each other.
Here are some key differences:
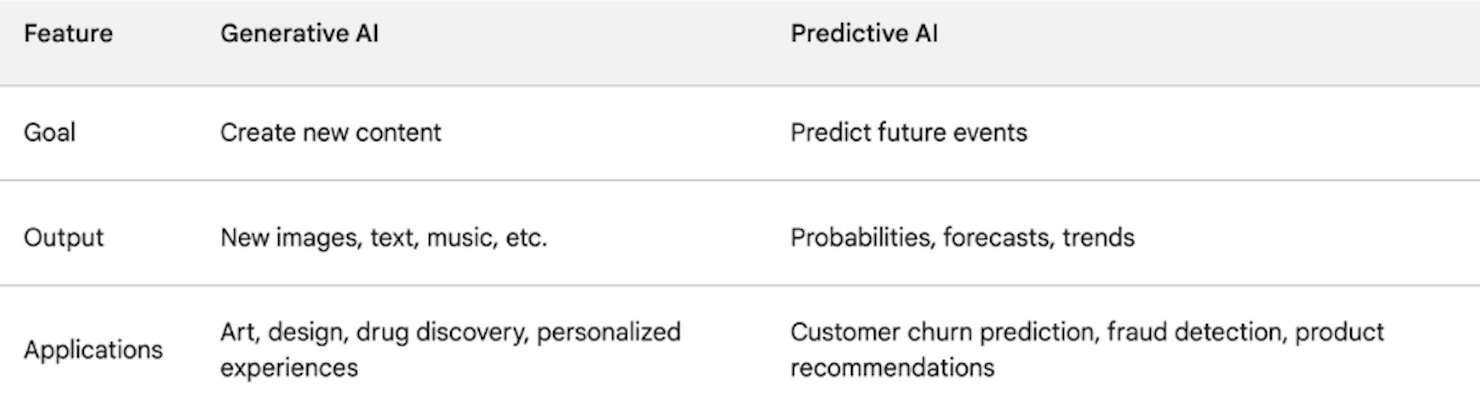
Generative AI
Generative AI focuses on creating new and original content. Think of it as a digital artist or musician, taking inspiration from existing data and styles to produce something entirely new.
Generative AI works by learning the underlying patterns and relationships within data. It then uses this knowledge to create new data that aligns with those patterns but is also unique and original. This opens up a vast range of possibilities, from revolutionizing creative industries to accelerating scientific discovery.
Predictive AI
Predictive AI, on the other hand, is all about forecasting future events. It's like a fortune teller with a Ph.D. in statistics, analyzing massive amounts of historical data to identify patterns and trends.
Predictive AI relies on complex algorithms and statistical models to analyze data and make predictions. While it can't tell you the future with absolute certainty, it can provide valuable insights that can help individuals and organizations make better decisions.
In short, generative AI is about creating, while predictive AI is about predicting. They both use powerful AI techniques, but they have different goals and applications. As AI continues to evolve, both generative and predictive AI are poised to play an increasingly important role in our lives.
How Generative AI Works
Generative AI uses neural networks and deep learning to generate new data, like images, text, or speech. It works by analyzing a huge amount of data to find patterns and learn how certain types of content can be created.
Once the neural network has learned from the data, it can generate completely new examples that mimic the style and form of the training data.
Generative AI powers things like:
- Image generation: Creating new photos of people, animals, objects, and scenes. Systems like GANs (generative adversarial networks) can generate highly realistic images.
- Text generation: Producing new articles, stories, or social media posts in a particular style. OpenAI's GPT-3 is an example of a large language model that can generate articles and create social media content.
- Music generation: Coming up with new melodies, harmonies, and even entire songs in a chosen genre or style. Researchers have developed AI systems that can generate pop songs, classical music, and more.
- Speech generation: Creating artificial speech using neural networks trained on thousands of hours of human speech data. Systems like Google's DeepMind can generate natural-sounding speech.
The key to generative AI is having huge amounts of data to train the neural networks on. The more data the AI has to learn from, the better it can identify patterns and "understand" how to generate new examples. Generative AI uses those patterns to create new data that resembles the style, form, and quality of the training data.
How Predictive AI Works
Predictive AI uses machine learning to make predictions about future outcomes based on historical data. It works by analyzing patterns in past data to build a model that can predict future events or behaviors.
Predictive AI powers things like:
- Fraud detection: Using data about past fraudulent transactions to identify potentially fraudulent transactions in the future.
- Customer churn prediction: Analyzing customer data like purchase history and service usage to predict which customers are likely to cancel their subscriptions or stop using a service.
- Sales forecasting: Examining factors like past sales, seasonality, market trends, and customer behavior to predict future sales numbers.
- Risk assessment: Looking at data on past risks, failures, and issues to predict the likelihood and impact of future risks.
- Personalized recommendations: Analyzing a customer's past purchases and online behavior to suggest relevant product recommendations.
Like generative AI, predictive AI requires large amounts of historical data to train machine learning models. The more accurate and comprehensive the training data, the better the predictive model will be. Predictive AI uses patterns it identifies in past data to make educated guesses about what will happen in the future.
Advantages and Limitations of Generative AI
Generative AI has significant advantages over predictive AI for certain applications.
- Increased creativity and innovation: Generative AI can be used to create new and original ideas, which can lead to breakthroughs in various fields. For example, it can generate new product ideas, or create new forms of art.
- Automation of tasks: Generative AI can be used to automate repetitive tasks, which can free up human workers to focus on more creative and strategic work. For example, it can be used to generate marketing copy, write news articles, or create social media content.
- Personalization: Generative AI can be used to personalize content and experiences for individual users. For example, it can be used to recommend products, generate custom news feeds, or create personalized learning experiences.
However, generative AI also has some key limitations:
- Bias: Generative AI models can be biased, reflecting the biases that are present in the data they are trained on. This can lead to unfair and discriminatory outcomes.
- Lack of control: It can be difficult to control the outputs of generative AI models, which can lead to unintended consequences. For example, a generative AI model might generate fake news articles or create deepfakes that are difficult to distinguish from reality.
- Limited understanding: Generative AI models do not have a deep understanding of the world, which can limit their ability to generate truly creative and innovative content.
Advantages and Limitations of Predictive AI
Predictive AI has significant advantages over generative AI for certain applications.
- Enhanced Decision Making: Predictive AI provides insightful forecasts that help businesses make informed decisions. By predicting trends and customer behavior, companies can plan strategies with a higher degree of confidence.
- Risk Reduction: By anticipating potential issues before they arise, predictive AI can alert businesses to risks, allowing them to take proactive steps to mitigate them. This is particularly useful in financial services for credit scoring and fraud detection.
- Optimized Operations: Predictive AI can streamline operations by predicting maintenance needs in machinery and equipment. This predictive maintenance means less downtime and more efficient use of resources.
- Personalization: In marketing, predictive AI can analyze customer data to personalize experiences, recommending products or services tailored to individual preferences, which can enhance customer satisfaction and loyalty.
However, predictive AI also has some limitations:
- Bias: Predictive models can reflect and perpetuate historical biases in the data they are trained on. This can lead to unfair outcomes for underrepresented groups.
- Lack of explainability: The inner workings of complex predictive models can be difficult for humans to interpret and explain. This can reduce user trust in the predictions.
- Overfitting: Predictive models are at risk of overfitting to historical training data, which can reduce their ability to generalize to new data.
- Ethical concerns: There are ethical concerns around the use of predictive models to make sensitive decisions that impact people's lives. Strict guidelines, auditing, and transparency are needed.
Generative AI Applications
Generative AI is an area of artificial intelligence that focuses on creating new content, whether that be text, images, audio, or even video. It's a pretty exciting field because it's constantly evolving and finding new ways to apply the technology. Here are some applications:
1. Content Creation: AI can generate articles, stories, and even poetry. Tools like Hypotenuse AI can create coherent and contextually relevant text based on prompts given to them.

2. Art and Design: Generative AI can produce stunning visuals, from original artwork to graphic design elements. HypoArt can create images and art that are difficult to distinguish from those made by humans.
3. Music Composition: AI can now compose music in various genres, which can be used for background scores, video games, or even as standalone pieces.
4. Voice Generation: As we touched on earlier with Google's DeepMind, AI can generate speech that sounds incredibly lifelike, which has applications in virtual assistants, customer service bots, and more.
5. Personalized Marketing: AI can generate personalized advertising content tailored to individual preferences, potentially increasing engagement and conversion rates.
As you can see, generative AI is driving major advances in content, ecommerce, entertainment, transportation, and more. While narrow AI can perform specific, limited tasks, generative AI allows for more open-ended, creative applications that continue to push the boundaries of what’s possible with artificial intelligence. The future is bright!
Predictive AI Applications
Predictive AI has a wide variety of applications across industries. Here are some examples:
1. Customer Retention: Predictive models can determine which customers are at risk of churn based on their behavior patterns and characteristics. This allows companies to proactively reach out and improve customer loyalty.
2. Fraud Detection: AI can detect fraudulent transactions and activity in real-time by analyzing spending patterns and comparing them to known fraud indicators. This helps prevent losses from credit card fraud, insurance scams, and more.
3. Healthcare: In healthcare, predictive AI can forecast patient admissions and outcomes, and it's being used to develop personalized medicine strategies. It can also predict disease outbreaks and the spread of infections
4. Predicting Demand: Predictive AI models use historical data to forecast demand for products and services. This helps businesses optimize inventory levels, staffing, and supply chain management.
5. Personalized Recommendations: AI-powered recommendations of products, content, and offers can be tailored to individual customers based on their preferences and past behaviors. This improves customer experience and increases engagement.
The Future of Generative AI and Predictive AI

The future of generative and predictive AI holds immense potential to revolutionize industries by enhancing creativity, efficiency, and decision-making processes.
Generative AI, which is capable of creating new content, such as images, text, and music, is becoming increasingly sophisticated. According to Gartner, generative AI's impact on businesses will continue to expand dramatically in the next five years. Generative AI will considerably transform the work of employees, whether creating text, visuals, hardware designs, tunes, movies, or other things. In response, workers will have to evolve into content editors, requiring a distinct set of skills from content creation.
Predictive AI, on the other hand, is expected to become even more accurate and insightful. According to Forbes, the use of predictive AI is anticipated to become more prevalent in solving real problems as next-generation organizations have already harnessed the power of these technologies to generate remarkably accurate predictions
Overall, as AI technologies like generative and predictive models advance and integrate, the benefits for businesses and society as a whole will be tremendous. While there are challenges ahead, the future of AI promises to reshape our world in positive ways if implemented responsibly.
So, which type of AI is right for you?
It really depends on your needs. If you're looking for a tool to help you create new and innovative content, then generative AI is a great option.
One of these is Hypotenuse AI, an AI content writing tool that uses machine learning to generate human-like text. It can produce a wide range of content types, including blog posts, product descriptions, social media captions, and more.
Generative AI and predictive AI are two sides of the same fascinating tech coin. One helps you imagine and create while the other helps analyze patterns and predict outcomes. Both have huge potential to transform our lives and the world around us.
At the end of the day, AI in all its forms is still a tool. How we choose to apply that tool - whether to generate new ideas or gain useful insights - is up to us. The future is unwritten, and it's exciting to think of all the ways these emerging technologies might help us shape it for the better.